βπ, ππππ π₯π ππππ₯ πͺπ π¦!
My name is Jia Xu, and I was born in Wuxi, China. My passion for data science has led me to pursue a Data Analytics Master of Science in Social Policy degree (MSSPDA) at the University of Pennsylvania. Inspired by real-world problems in the fields of finance and health, I am driven to learn and apply data science and analytics skills to make a meaningful impact in these areas.
I currently hold positions as a research assistant at the Shen Lab, Perelman School of Medicine, where I focus on developing and applying data science methods to extract actionable insights from complex biomedical and health data. Additionally, I am a research assistant in the political science department at the University of Pennsylvania, working collaboratively with UC Berkeley and Columbia University to measure the pro/anti government bias of different TV stations using NLP techniques.
With an undergraduate degree in mathematics and applied mathematics and ongoing graduate studies in social policy and data analytics, I am passionate about leveraging my expertise in both health and business settings. My talent for analyzing complex data sets and my keen eye for detail have allowed me to develop a proven track record of success in various data science roles, from conducting intricate data analysis and modeling to designing innovative data-driven strategies and solutions.
Driven by my desire to make a positive difference in the world, I bring a unique combination of technical expertise and strategic vision to every project I undertake. Whether working in the healthcare industry to develop more effective treatments and therapies or partnering with businesses to optimize operations and drive growth, I am committed to leveraging data science for good.
I am passionate about learning and continuously seek new opportunities to challenge myself and apply my skills to drive meaningful outcomes. Thank you for taking the time to visit my personal website, and I look forward to exploring how I can contribute to your team or organization.
M.S. Data Analytics in Social Policy β University of Pennsylvania β 2023
B.S. in Mathematics and Applied Mathematics β Nanjing University of Finance and Economics β 2021
What I’m up to lately
Published Works
Clustering Alzheimerβs Disease Subtypes via Similarity Learning and Graph Diffusion
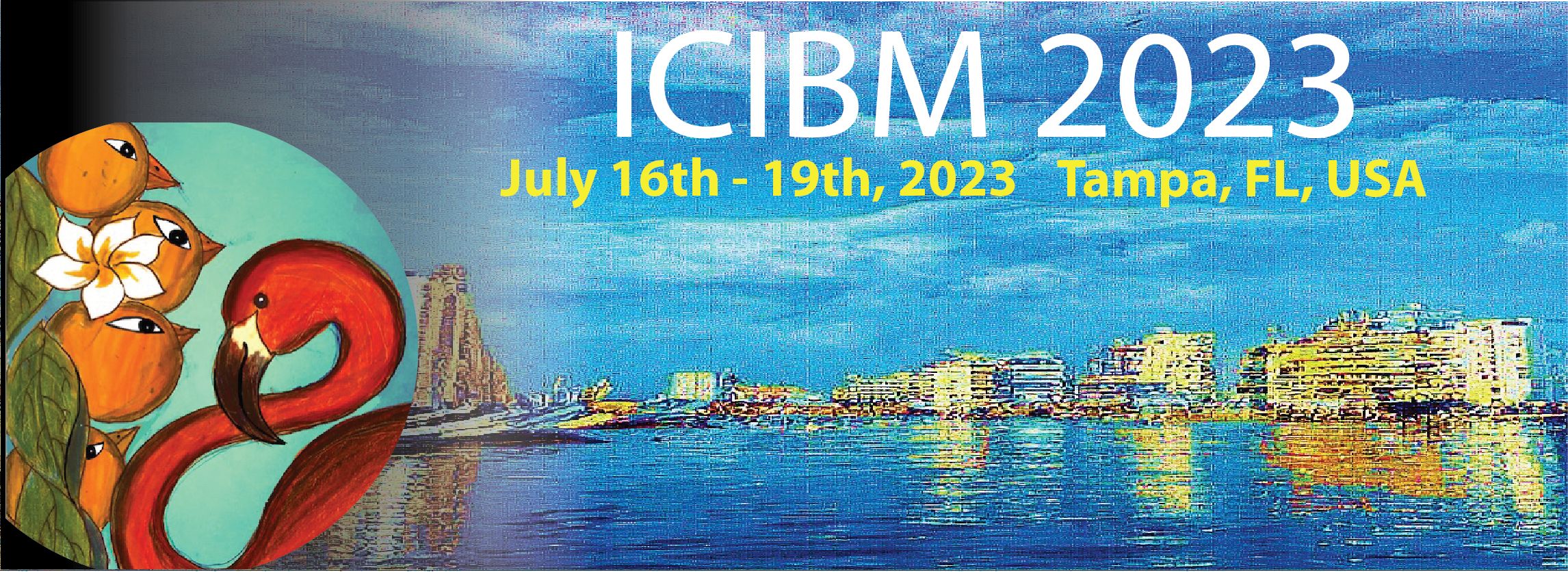
Alzheimer’s disease (AD), a complex neurodegenerative disorder affecting millions globally, presents diagnostic and treatment challenges due to its heterogeneous nature. This study targets the identification of homogeneous AD subtypes with unique clinical and pathological characteristics to overcome these issues. Using an innovative approach with unsupervised clustering, the multi-kernel similarity learning framework SIMLR, and graph diffusion, we analyzed MRI-derived cortical thickness measurements in 829 patients with AD and mild cognitive impairment (MCI). The unique clustering methodology we used, untested in AD subtyping before, outperformed traditional clustering methods, notably mitigating noise interference in subtype detection. Five distinctive subtypes emerged, differing significantly in biomarkers, cognitive status, and other clinical features. A subsequent genetic association study validated these subtypes, uncovering potential genetic connections. Read more
Courses I've taken so far
CIS 545: Big Data Analytics
In the era of big data, we are increasingly faced with the challenges of converting massive amounts of data to actionable knowledge. Given the limits of individual machines (compute power, memory, bandwidth), increasingly the solution is to clean, integrate, and process the data using statistical machine learning techniques, in parallel on many machines. This course focuses on the fundamentals of scaling computation to handle common data analytics tasks. You will learn about basic tasks in collecting, wrangling, and structuring data; programming models for performing certain kinds of computation in a scalable way across many compute nodes; common approaches to converting algorithms to such programming models; standard toolkits for data analysis consisting of a wide variety of primitives; and popular distributed frameworks for analytics tasks such as filtering, graph analysis, clustering, and classification. Read more
My Interest
Fitness
η±θͺε·±οΌζ―η»θΊ«ζ΅ͺζΌ«ηεΌε§γ Read more
My Past Projects
Uncovering Inequities in Green Space (Green Space Data Challenge)
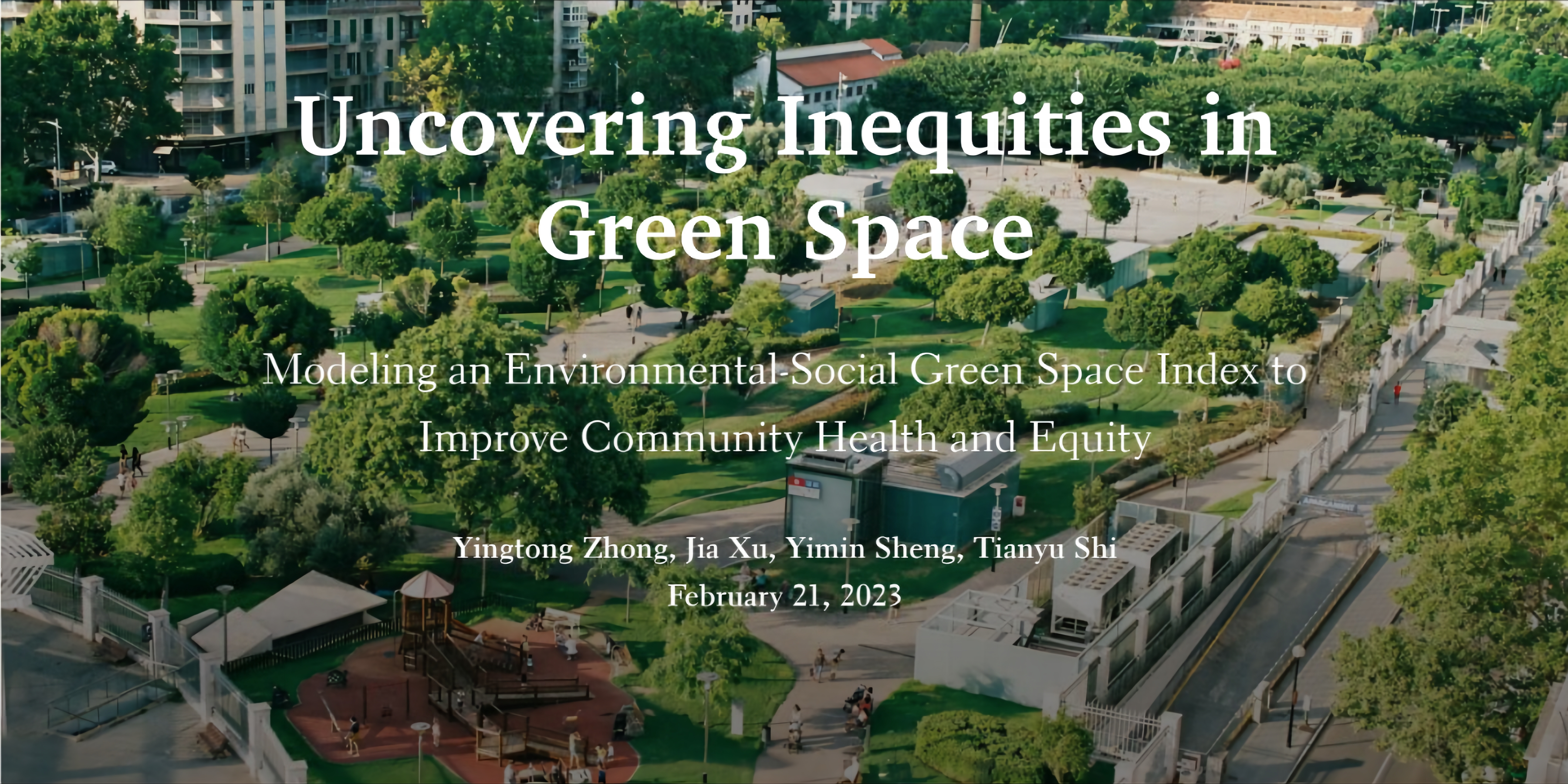
With the rapid growth of urbanization, green spaces in the form of parks, gardens, and other open areas are extremely important for improving the quality of life for those who live in urban areas. As polluted air and water and overcrowded cities become our everyday lives, the physical and mental health of urban communities has undoubtedly gained increasing attention in recent years. Not surprisingly, green spaces are coming to the rescue. Cancel changes Open spaces such as parks benefit communities by providing a cleaner environment to protect public health such as a reducing in the urban heat island effect and improving air quality. They can also have an impact on social health by contributing to increase community cohesion and wellbeing, and increased property values, among other positive environmental, social, and financial outcomes. As a result, the supply of green space and the ease with which it can be accessed are key concerns in urban planning and policy-making. Read more
Featured categories
Python (11) Machine Learning (7) Data Science (4)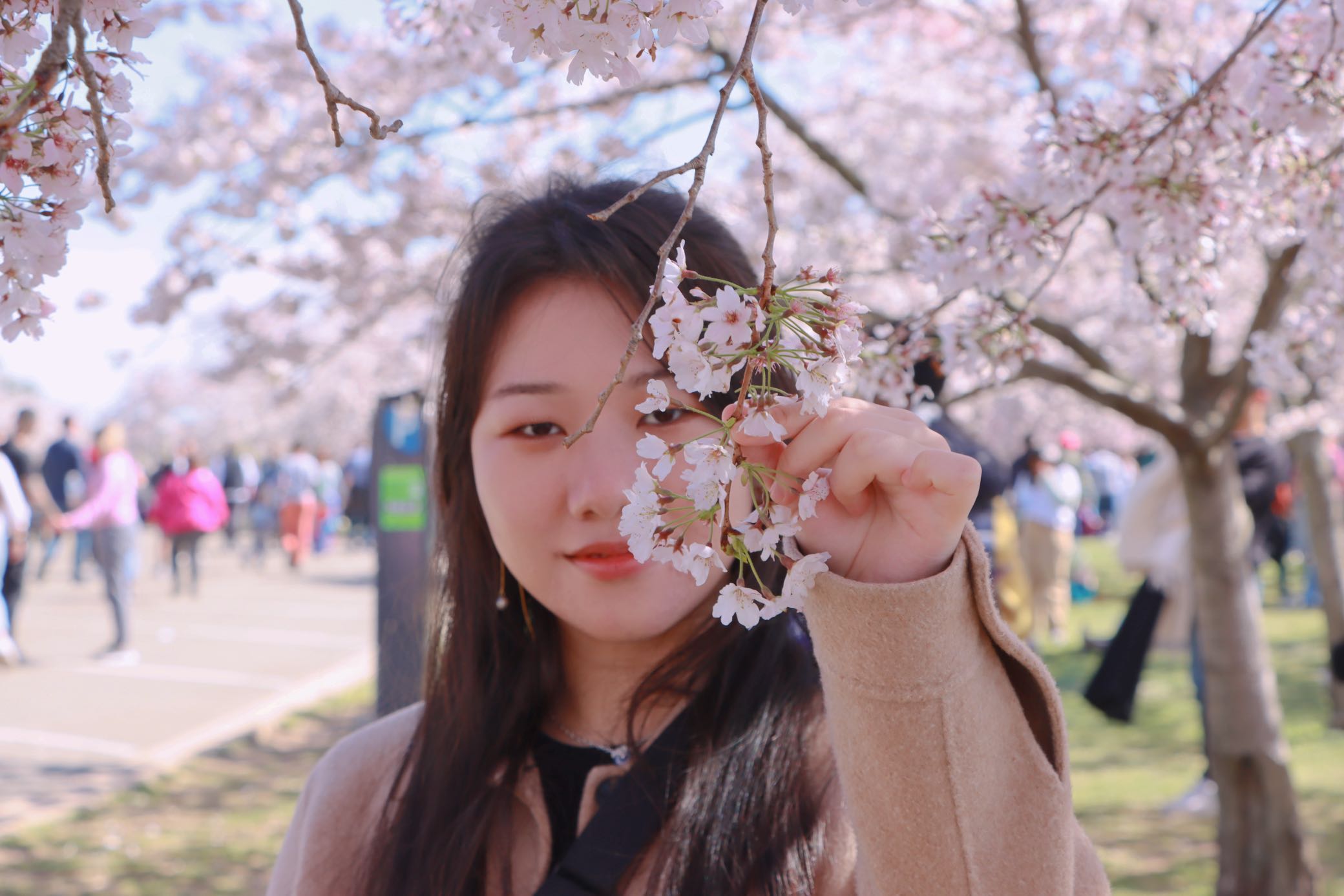
Jia Xu
Graduate Student @ UPenn
How to say my name